SiW-Mv2 dataset contains 14 spoof types spanning from typical print and replay attack, to various masks, impersonation makeup and physical material coverings. SiW-Mv2 has the largest variance in terms of the spoof pattern, each of these patterns are designated and verified by the IARPA project. For example, obfuscation makeup and partial coverings intend to hide subject’s identity; impersonate and 3 mask modifies the subject appearance for imitating other identities. SiW-Mv2 collects 785 videos from subjects from 493 subjects, and 915 spoof videos from 600 subjects. The details of each categories are in the follow images. More details can be found at link.
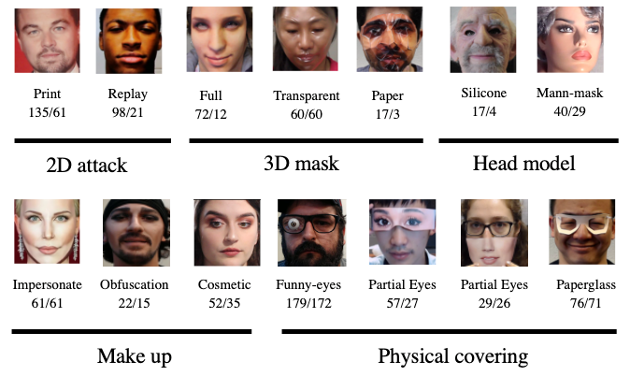
Evaluation Protocols
We offer three protocols and the baseline performance (more details):
We split live subjects and subjects of each spoof pattern into train and test splits. Given such training and test partition files, we evaluate our model and report the performance. This protocol evaluates the model capability of detecting the known spoof pattern.
We use the leave-one-out paradigm, which keeps 13 spoof pattern samples in the training, and one remaining spoof pattern for the spoof section evaluation. This protocol evaluates the model capability of detecting the unknown spoof pattern.
We follow the attribute label (e.g., spoof pattern, race, age and illumination), to evaluate the model performance on the unseen target domain. This protocol evaluates the model generalization ability.
Download
Our SiW-Mv2 database is available under a license from Michigan State University for research purposes. Sign the Dataset Release Agreement (DRA) and send to guoxia11@msu.edu. You will receive the download username/password and instructions upon approval of your usage of the database, and you can download SiW-Mv2 database within 30 days from approval.