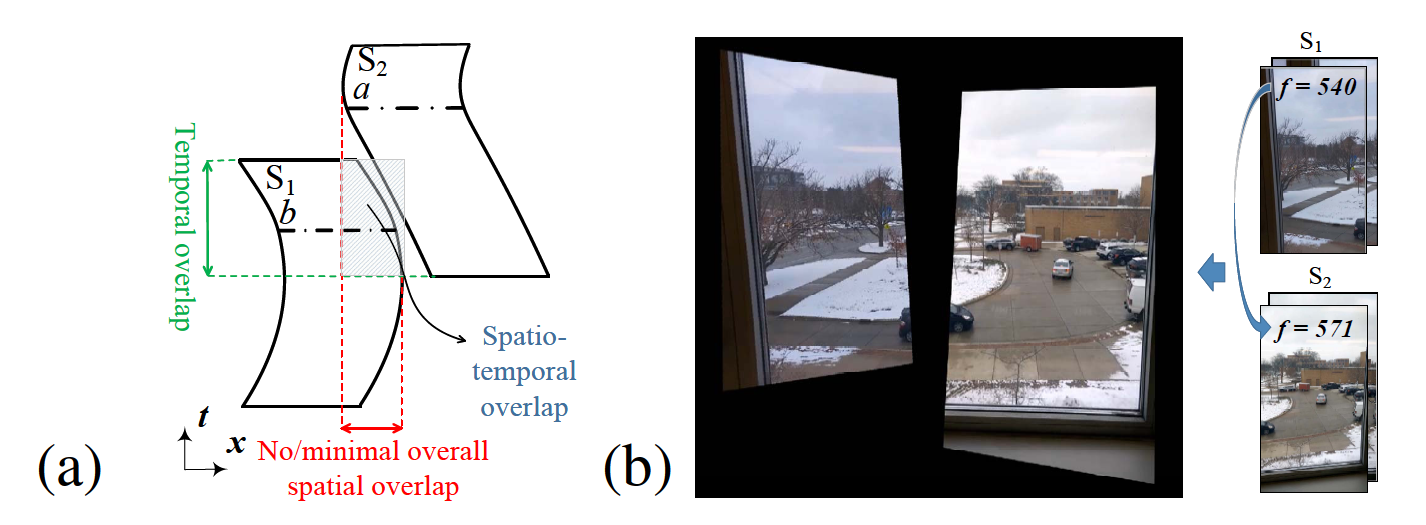
Spatio-Temporal Alignment of Non-Overlapping Sequences from Independently Panning Cameras
Seyed Morteza Safdarnejad, Xiaoming LiuWe tackle a novel scenario of spatio-temporal alignment of seuqences referred to as Nonoverlapping Sequences (NOS). NOS are captured by multiple freely panning handheld cameras whose field of views (FOV) might have no direct spatial overlap. With the popularity of mobile sensors, NOS rise when multiple cooperative users capture a ...
Continue readingKeywords: Motion Compensation, activity recognition