The prevalence of facial recognition, biometric unlock, and social media presents a significant opportunity for bad actors to introduce forged or manipulated images to spread false information or damage reputations. This is aided by the continuing improvement in realistic image synthesis and manipulation by generative adversarial network, GAN, based methods. The DFFD dataset combines multiple forgery types in a single dataset.
Dataset Description
The DFFD dataset is comprised of multiple publically available datasets and images that are synthesized/manipulated using publically available methods. By incorporating multiple sources for real images, we are able to include varying resolution and image quality for both real and synthetic/manipulated images. More details are in Section 4 of our paper.
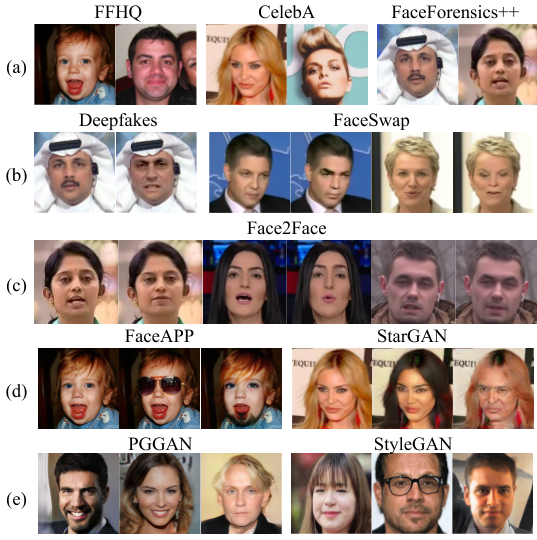
Figure 1: Sample images in the DFFD dataset.
Evaluation Protocol
We split the DFFD dataset into training (50%), validation (5%), and testing (45%) partitions, while taking care to enforce that an identity in one partition does not appear in any other partition. While training, when we observe convergence of the model on the validation partition, we freeze the model and evaluate on the testing partiion.
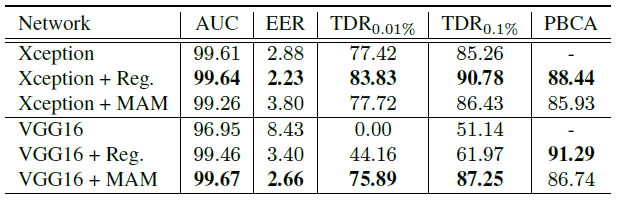
Figure 2: The baseline performance on the DFFD dataset.
How To Download
1. DFFD dataset is available for research purposes. We provide images that we are able to, and instructions for images that we cannot release ourselves.
2. Submit the request at Online Application.
3. If you are unable to access Google Server in Step 2, please send an email to dffd.dataset@gmail.com with the following information:
- Title: DFFD Application
- CC: Your advisor's email
- Content Line 1: Your name, email, affiliation
- Content Line 2: Your advisor's name, email
- Content Line 3: Research Lab Webpage (Wrong formats may require longer time to process!)
4. You will receive the download password and instructions upon approval of your usage of the dataset, and you can download the DFFD dataset.
Acknowledgements
-
On the Detection of Digital Face Manipulation
Hao Dang*, Feng Liu*, Joel Stehouwer*, Xiaoming Liu, Anil Jain
In Proceeding of IEEE Computer Vision and Pattern Recognition (CVPR 2020), Seattle, WA, Jun. 2020
Bibtex | PDF | arXiv | Project Webpage