Gait, the walking pattern of individuals, is one of the most important biometrics modalities. Most of the existing gait recognition methods take silhouettes or articulated body models as the gait features. These methods suffer from degraded recognition performance when handling confounding variables, such as clothing, carrying and view angle. To remedy this issue, we propose a novel AutoEncoder framework to explicitly disentangle pose and appearance features from RGB imagery and the LSTM-based integration of pose features over time produces the gait feature. In addition, we collect a Frontal-View Gait (FVG) dataset to focus on gait recognition from frontal-view walking, which is a challenging problem since it contains minimal gait cues compared to other views. FVG also includes other important variations, e.g., walking speed, carrying, and clothing. With extensive experiments on CASIA-B, USF and FVG datasets, our method demonstrates superior performance to the state of the arts quantitatively, the ability of feature disentanglement qualitatively, and promising computational efficiency.
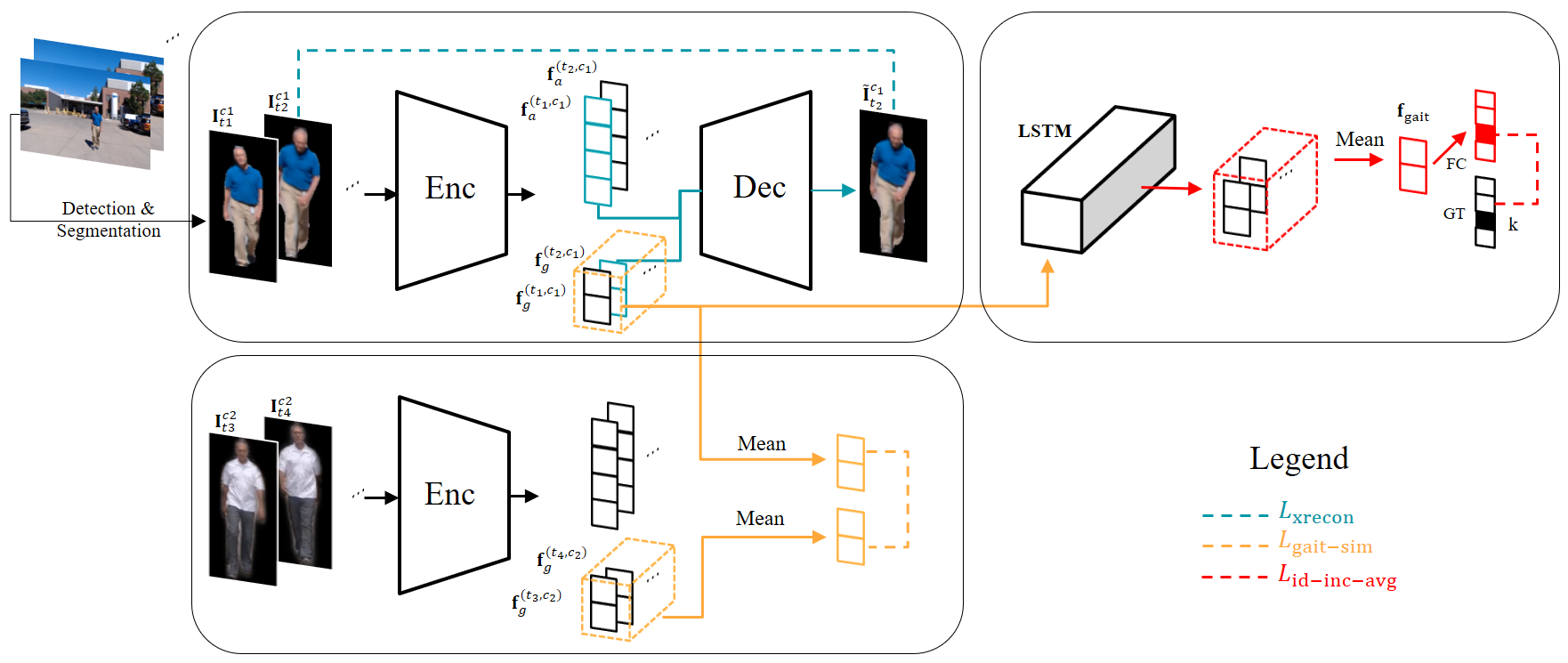
Figure 1: Overall architecture of our proposed approach, with three novel loss functions.
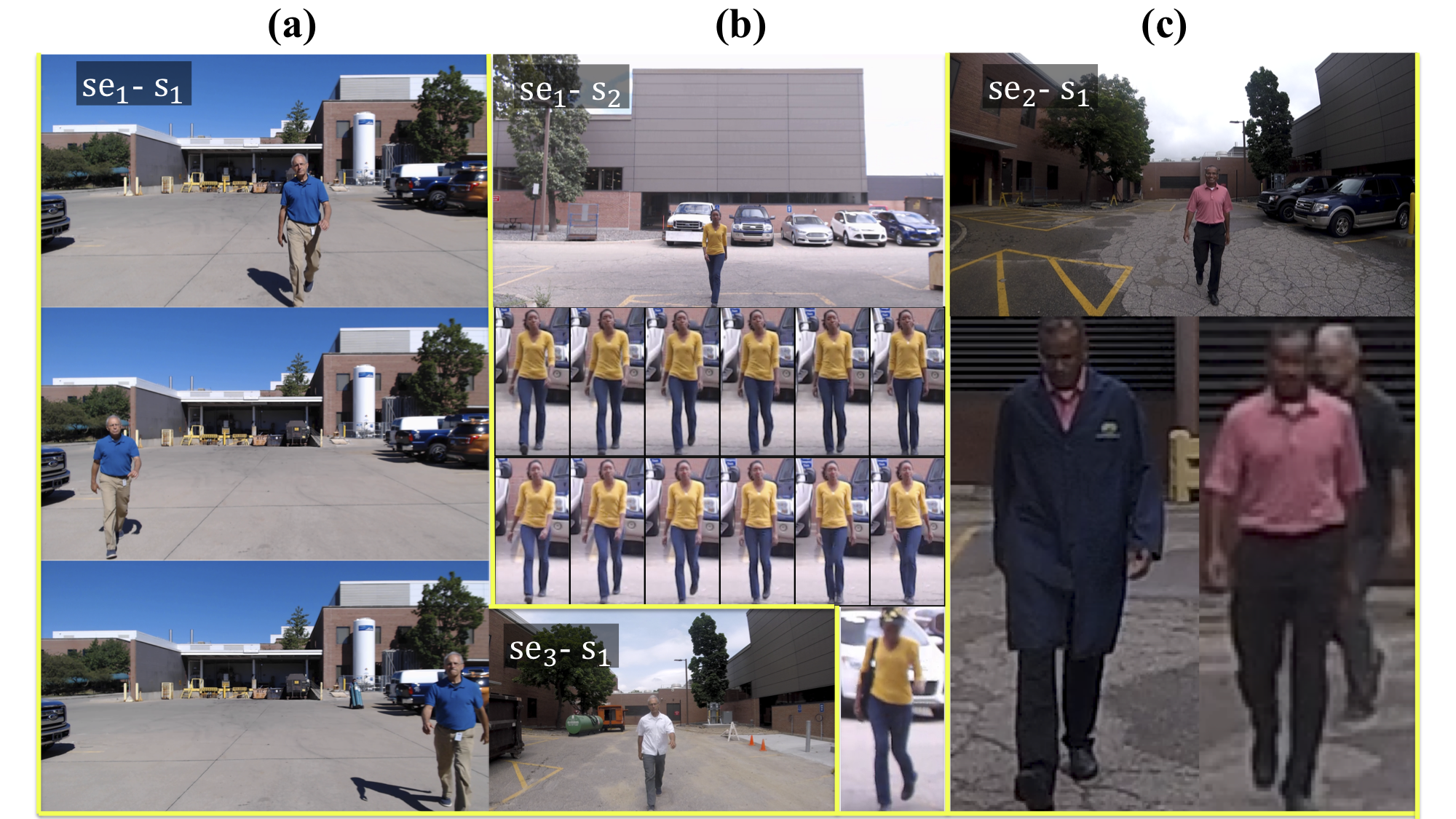
Figure 2: Examples of FVG Dataset. (a) Samples of the near frontal middle, left and right walking view angles in session 1 (SE1) of the first subject (S1). SE3-S1 is the same subject in session 3. (b) Samples of slow and fast walking speed for another subject in session 1. Frames in top red boxes are slow and in the bottom red box are fast walking. Carrying bag sample is shown below. (c) samples of changing clothes and with cluttered background from one subject in session 2.
FVG Database
FVG database download page: http://cvlab.cse.msu.edu/frontal-view-gaitfvg-database.html
GaitNet Source Code
The source code for the networks is available at: https://github.com/ziyuanzhangtony/GaitNet-CVPR2019